Generative AI: short for Generative Artificial Intelligence
2 min read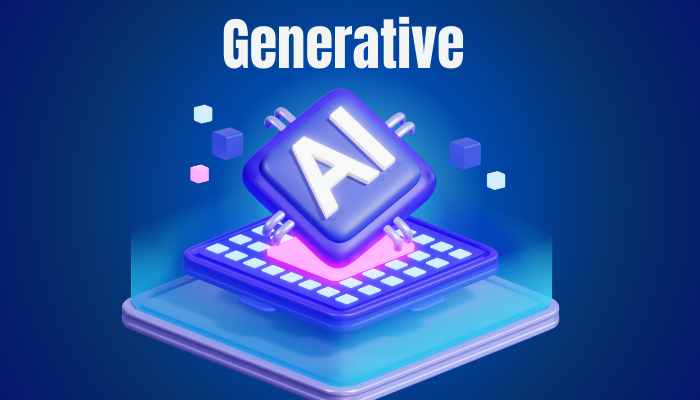
Generative artificial intelligence, or generative AI for short, is a class of artificial intelligence systems that are intended to produce new material, frequently in the form of writing, photographs, or other data. These systems use sophisticated algorithms, such as deep learning models, to recognise and reproduce patterns in the input data, producing unique and frequently lifelike results. Key components of generative AI are as follows:
- Generative Models:
- Definition: Generative models are a type of machine learning model that learns the underlying patterns of a dataset to generate new, similar data. They contrast with discriminative models, which focus on classifying and distinguishing between different data categories.
- Types of Generative AI:
- Generative Adversarial Networks (GANs): GANs consist of two neural networks, a generator and a discriminator, that are trained simultaneously. The generator creates data, and the discriminator evaluates its authenticity. The competition between the two networks results in the generation of increasingly realistic content.
- Variational Autoencoders (VAEs): VAEs are another type of generative model that aims to encode and decode input data. They learn a probabilistic mapping between the input and a latent space, allowing the generation of new data points.
- Applications:
- Image Generation: Generative AI is widely used to create realistic images, often indistinguishable from photographs. This has applications in art, design, and even the generation of synthetic data for training machine learning models.
- Text Generation: Natural Language Processing (NLP) models based on generative AI can create coherent and contextually relevant text. This is applied in chatbots, content creation, and even creative writing.
- Style Transfer: Generative models can transfer the style of one image to another, allowing for artistic transformations and visual effects.
- Creative Applications:
- Art and Design: Generative AI has been employed in various artistic and design projects, creating unique pieces of visual art, music, and other creative works.
- Procedural Content Generation: In the gaming industry, generative AI is used to create dynamic and varied game environments, characters, and narratives.
- Ethical Considerations:
- Deepfakes: The ability of generative AI to create highly realistic content has raised concerns, particularly with the creation of deepfake videos where faces or voices are convincingly manipulated.
- Bias in Data: Generative models may inadvertently perpetuate biases present in the training data, leading to biased outputs.
- Research Challenges:
- Training Stability: Achieving stable training for generative models, especially large-scale ones like GANs, is an ongoing research challenge.
- Interpretable Outputs: Understanding and interpreting the decisions made by generative AI models, especially in complex tasks like image synthesis, remain areas of active research.
Generative AI is a rapidly evolving field with both creative and practical applications. As it continues to advance, researchers are addressing challenges and exploring new ways to harness its potential while ensuring ethical and responsible use.